The trade-off every AI company will face
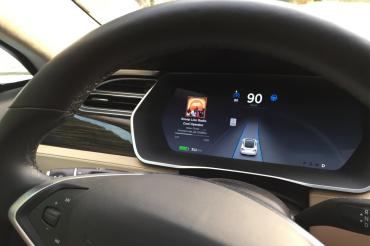
Published: March 29, 2017
A new op-ed by U of T's Ajay Agrawal, Joshua Gans and Avi Goldfarb in the Harvard Business Review examines the trade-offs faced by AI companies like those creating self-driving cars.
Artificial intelligence (AI) applications are based on generating predictions, and machine learning involves algorithms evolving through various learning processes, they write. As a machine is given data, including outcomes, it finds associations, and then, based on those associations, it takes new data it has never seen before and predicts an outcome.
The trio, who are all affiliated with the Rotman School of Management, argue that this means that intelligent machines need to be trained, and that there are no ready-made answers as to what is “good enough” for machine intelligence.
“Instead, there are trade-offs,” they write. “Success with machine intelligence will require taking these trade-offs seriously and approaching them strategically.”
Agrawal is a professor of entrepreneurship and founder of the Creative Destruction Lab. Gans is a professor of strategic management, and Goldfarb is a professor of marketing.
Read more at the Harvard Business Review